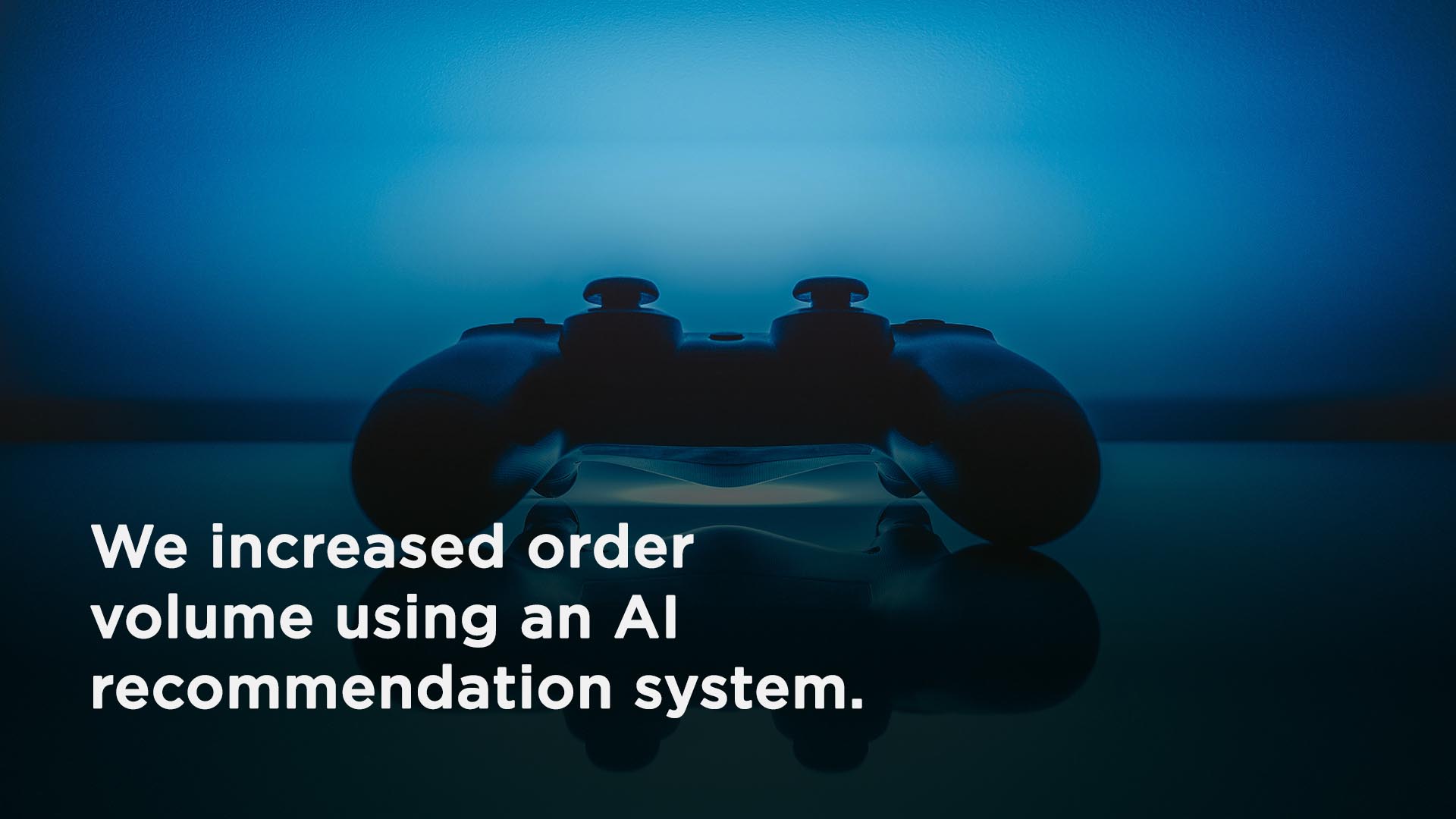
Key Challenges
• Refine data to determine design attributes and customer attributes.
• Train a recommendation system to determine the most common designs and components.
Business Case
Because of the large number of possible color combinations, buyers often struggle to finalize purchases. MAQ Software created a product recommendation solution that recommends controller designs based on the number of times a custom design is saved by other buyers.
Solution
MAQ Software generated order recommendations based on common traits amongst saved designs using Azure Matchbox Recommender. (Matchbox Recommender is a probabilistic model for generating personalized recommendations of items to users of a web service.)Key Highlights
• Collected and refined usage statistics data from Omniture source.
• Trained Azure Matchbox Recommender system.
• Created interactive recommendation report based on Matchbox results.
First, we collected website data from the Omniture data statistics source. The data included visitor location, visit frequency, and item attributes visitors saved. Next, we refined the data, narrowing the results to specific designs and the save frequency of each design. Each design was rated according to how many times it was added to a customer cart.
Next, we used the refined data to train the Matchbox recommendation system to determine which customizations were most common. Finally, we outputted the Matchbox recommendations to the interactive report shown in Figure 1.
![]() |
Figure 1: Purchase recommendations report |
Business Outcome
Using Azure Matchbox Recommender, the recommendation engine suggests two similar designs after making each component choice. Buyers can still make their own fully customized designs, but the recommendations streamline the process by showing popular color combinations. The recommendations allow customers to make faster selections, making the purchasing process more enjoyable.Outcome Highlights
• Streamlined the purchasing process by identifying popular color combinations based on the buyers’ preferences.
• Increased ordering success by simplifying customization decision.
Click here to learn more about our AI expertise.