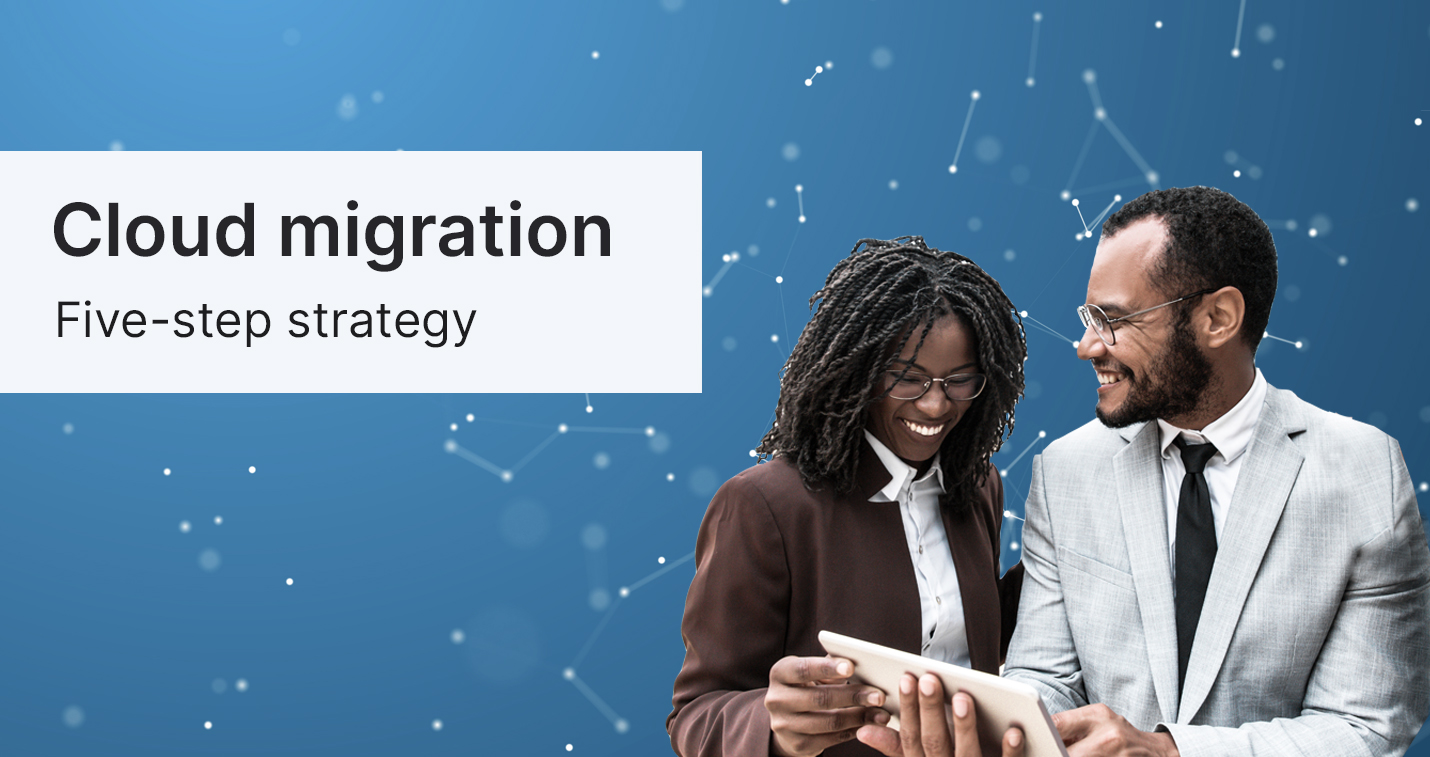
The Five Phases of Data Migration
Most modern businesses rely on data to generate insight, drive sales, and increase revenue. Migrating data systems to the cloud offers many benefits. Cloud architectures improve refresh speed, consolidate resources, and improve data uniformity. But for companies with terabytes, or even petabytes, of data, migrating to the cloud can seem challenging. Data migration also requires two production environments to run in parallel. Carefully navigating multiple logistical challenges requires a deeply considered plan.
We have over a decade of experience enabling cloud migrations for Fortune 500 companies. In this article, we will share the data migration process we have spent years refining. Our process is divided into five phases:
1.
Discovery
2.
Analysis and Design
3.
Planning and Approval
4.
Execution
5.
Verification
1. Discovery
During the discovery phase, we evaluate our client’s existing data system to understand their specific requirements. We examine data, sources, size, scale, refresh frequencies, and source relationships.
2. Analysis and Design
We divide our analysis and design phase into three steps:
1.
Research potentially applicable technologies
2.
Select the top technologies
3.
Perform a series of proofs of concept to determine technology suitability, cost benefit, and comparative features
3. Planning and Approval
During the planning and approval phase, we propose our solution and receive client feedback. Example: our most recent client tasked us with resolving four primary challenges:
1.
High data refresh and process times
2.
Server dependencies among multiple streams
3.
Difficulty managing and maintaining infrastructure
4.
Difficulty patching infrastructure
After analyzing the best options for this client, we presented the solutions that resolved their specific challenges:
1.
Implemented real-time data refresh pipelines for real-time reporting
2.
Improved processing speed by using the cloud’s distributed processing power
3.
Implemented a real-time job and asset monitoring framework
4.
Eased infrastructure maintenance by using the cloud’s serverless, scalable, and distributed architecture
5.
Enabled data publishing based on source availability using an intelligent job monitoring and validation framework
If you want to read more about how we enabled our client’s move to the cloud, check out our case study on cloud migrations.
4. Execution
During the execution phase, we implement the approved architecture. We determine which technologies to use for storage, process, publishing, and visualization.
•
Storage example: Azure Data Lake Storage
•
Process example: Azure Databricks
•
Publishing example: tabular models, SQL
•
Visualization example: Power BI, Excel
We then divide the project into seven steps:
1.
Stage upstream data
2.
Transfer the data
3.
Process the data
4.
Store the processed data in the cloud for downstream users to create reports
5.
Perform independent processing and refreshes using an intelligent job monitoring/ validation framework
6.
Process the tabular model (if applicable)
7.
Visualize reports
5. Verification
During the verification phase, we conduct user acceptance testing (UAT) through numerous user acceptance sessions. UAT allows technical users, business users, and operations team members to become familiar with the new data system as we gradually move the old system offline.
Benefits of Cloud Data Migration
Migrating to the cloud reduces data latency and improves data availability. Cloud-based data offers the latest data infrastructure and real-time reporting benefits. Centralizing data from numerous sources enables business teams to easily access and scale their reporting. Data can be processed more quickly and, in case of disaster, recovered easily with geo-replication. In addition, cloud infrastructures reduce data costs; maintenance is cheaper and easier to scale up or down based on company needs.
Our cloud data migration process effectively overcomes logistical challenges while achieving client objectives.
Do you want to migrate your company’s data to the cloud? Find out more about our cloud transformation expertise, or contact our sales team at Sales@MAQSoftware.com