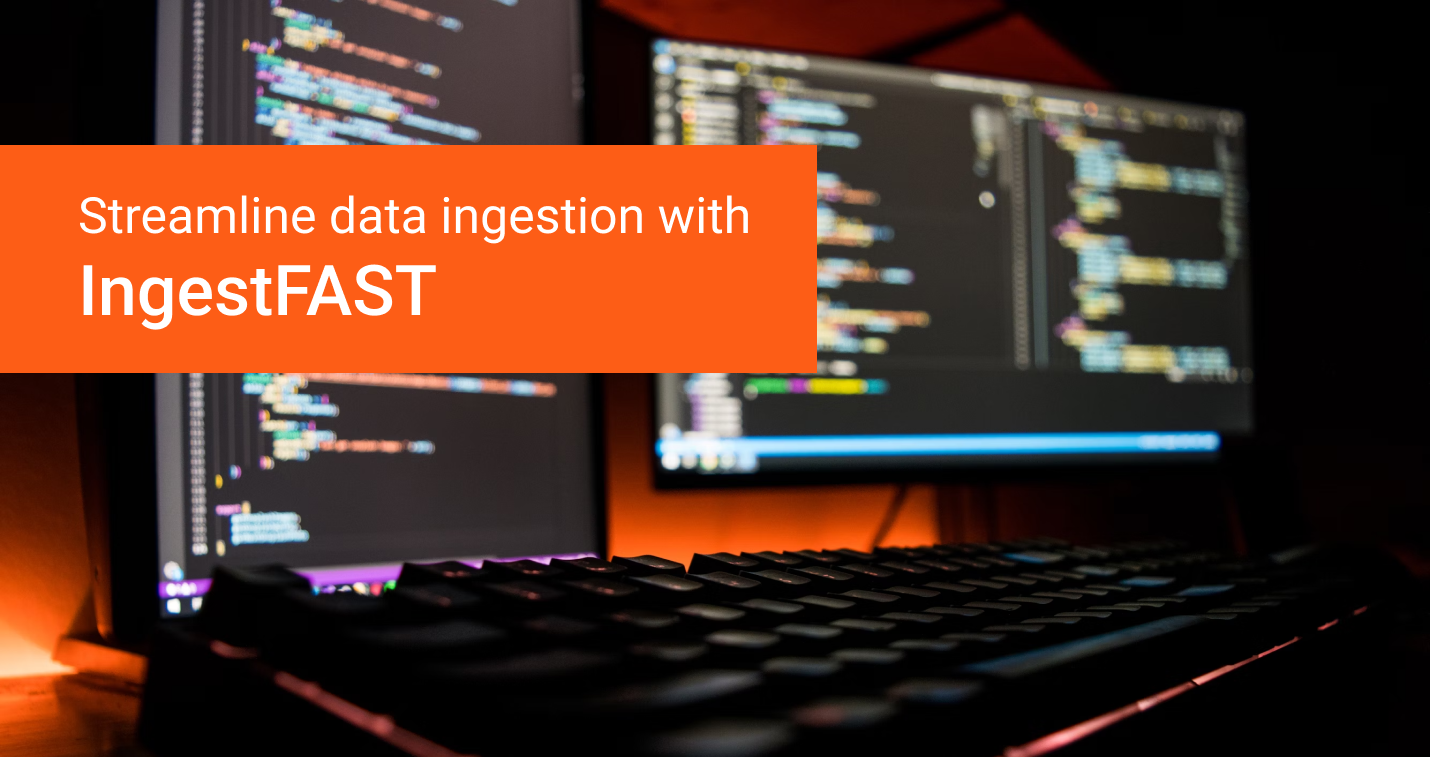
In today's data-driven world, organizations rely heavily on ingesting data from various sources to drive insights and make informed decisions. However, the process of ingesting data can be complex and challenging, involving multiple steps such as creating pipelines, defining pipelines, adhering to naming conventions, and monitoring data flow. This is where IngestFAST comes in, offering a comprehensive solution to streamline the data ingestion process and alleviate common challenges faced by organizations.
Challenges resolved by IngestFAST:
The data ingestion process can be complex, involving multiple steps and configurations.
Ensuring consistency across the data ingestion process can be challenging, especially in large organizations.
Monitoring data flow and troubleshooting issues can be time-consuming and challenging.
As data needs grow, scaling the data ingestion process can be difficult.
Key features of IngestFAST:
IngestFAST is based on a Master Template that defines parameters and creates configuration files for data ingestion. This template ensures consistency and standardization across the ingestion process, making it easier to manage and maintain.
With IngestFAST, users can quickly create pipelines for ingesting data from various sources. The framework automates the process of pipeline creation, saving time and effort for users.
IngestFAST provides robust monitoring capabilities that allow users to track the data flow in real time. This helps in identifying and resolving issues quickly, ensuring smooth data ingestion.
IngestFAST is designed to be highly scalable, allowing organizations to easily scale their data ingestion process as their data needs grow. The framework can handle large volume of data efficently, ensuring high performance.
While IngestFAST provides a standard framework for data ingestion, it also allows for customization to meet specific requirements. Users can tailor the framework to suit their unique data ingestion needs.
Key benefits of IngestFAST:
By automating the data ingestion process and providing monitoring capability, IngestFAST improves the efficiency of data ingestion, saving time and effort for organizations.
IngestFAST's Master Template-based approach ensures standardization across the data ingestion process, making it easier to manage and maintain.
While providing a standardized framework, IngestFAST also offers flexibility for customization to meet specific requirements, making it suitable for a wide range of use cases.
IngestFAST's scalability features allow organizations to easily scale their data ingestion process as their data needs grow, ensuring high performance.
Key use cases of IngestFAST:
Ingesting data from numerous third-party sources, such as social media platforms, customer relationship management (CRM) systems, and financial data providers.
Building and maintaining real-time data pipelines for applications like fraud detection, stock market analysis, and Internet of Things (IoT) data processing.
Ensuring consistent data ingestion practices across different departments or teams within an organization.
Streamlining data ingestion processes within cloud environments like Microsoft Azure Fabric.